Predictive maintenance aims to improve the profitability of the company by identifying problems that threaten the smooth running of the entire production chain.
This article aims to introduce you to a true flagship of Industry 4.0.
Predictive maintenance, a performance accelerator
Predictive maintenance is a modern industrial maintenance technique. moderne. Its aim is to go beyond-monitoring the condition ofa factory’s facilities. It helps to anticipate problems and identify symptoms. More agile than preventive maintenance, this new approach tends to take hold in many industrial sectors.
When preventive maintenance aims to anticipate failures by planning regular-interval control activities in advance (like your vehicle’s overhaul), predictive maintenance takes into account the data which are specific to each installation, in real time and continuously. This avoids unnecessary expenses such as replacements of spare parts scheduled at regular intervals.
“Anticipation” is the key word in predictive maintenance.
With predictive maintenance, it is now possible to capture machine data in real time and analyze it. This improves profitability but also improves customer satisfaction.
Valuable for equipment maintenance, predictive maintenance is primarily a way to avoid an unexpected shutdown of the production line. According to Intrasens, this technology reduces equipment downtime by more than 50% and equipment investments by 3% to 5%. Predictive maintenance is therefore synonymous with agility and savings.
A progressive learning process
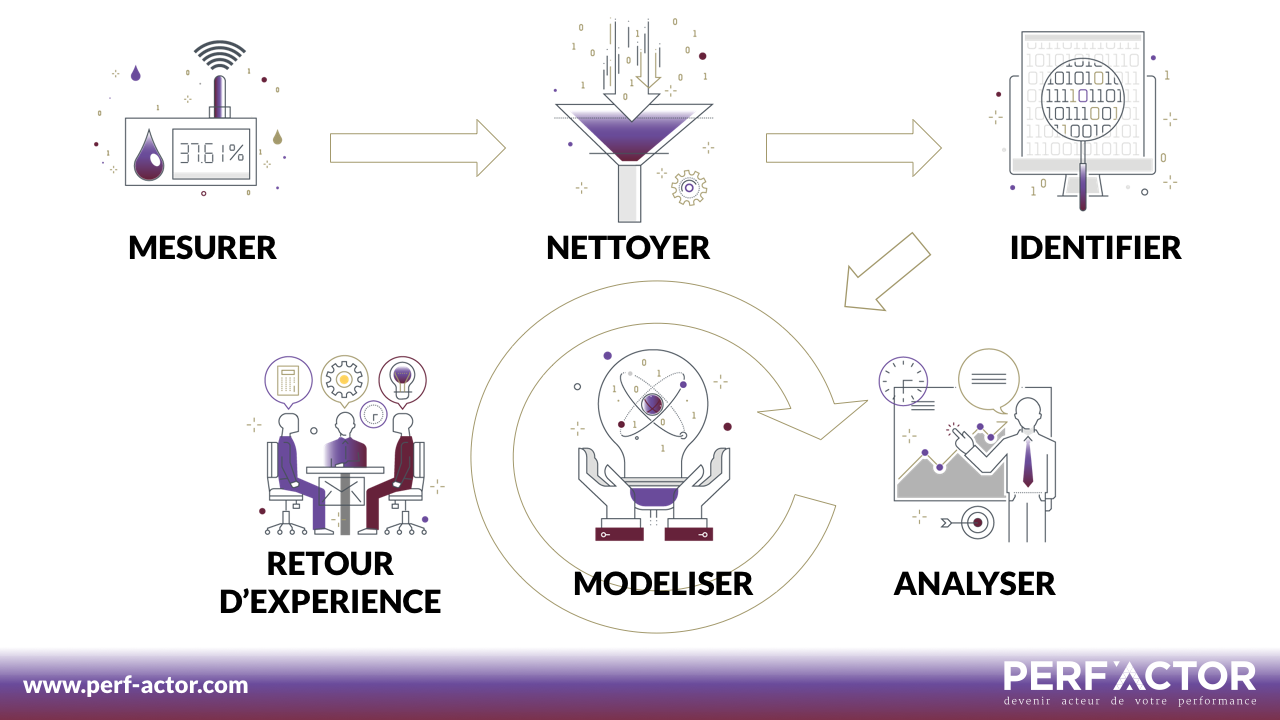
Step 1: Measuring information
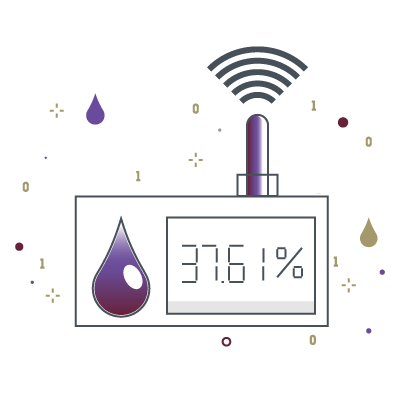
In order to collect data on an ongoing basis, the facilities will need to be equipped with sensors. These sensors allow the information to be collected in real time and transmitted to servers. The choice of these sensors will naturally depend on the type of installation.
Step 2 : Cleaning data
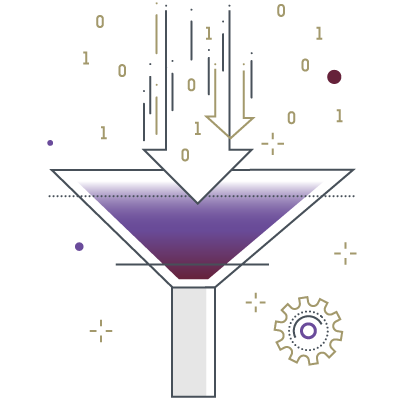
Raw data which are not converted into sensor output is very rarely usable as it is. There are many reasons for this: parasitic elements skew measurements on an ad hoc or permanent basis, duplicates may appear… It is therefore essential to filter and sort the data so that it can be used. Only data that is relevant from a predictive maintenance perspective should be selected.
Step 3 : identifying trends
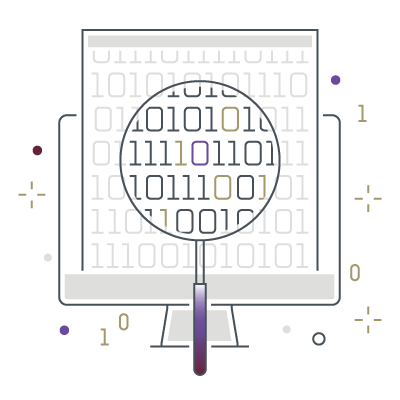
Once the data is cleaned, it must be analyzed to be able to identify trends with respect to the “normal” behavior of the installation or indications of the deterioration of this operation. The results of these analysis will lay the foundation for a predictive maintenance model for this installation.
Step 4: Modeling how it works
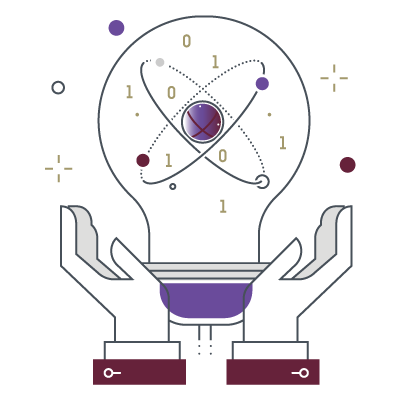
Your industrial installation will be modeled using specific algorithms and failure patterns.The degradation of the machines can therefore be anticipated by detecting the warning signals of more severe malfunctions. Real engine failures and production shutdowns can be avoided.
Step 5: Analyzing results
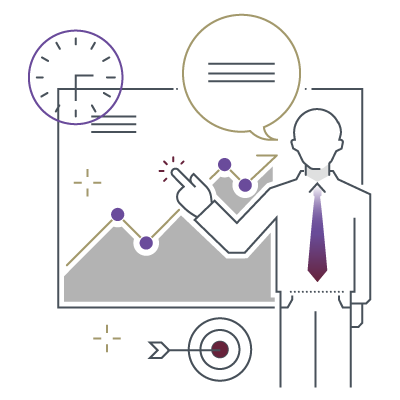
Predictive maintenance is based on the exploitation of historical data, so the results of the model must be regularly analyzed. This analysis makes it possible to propose recommendations to maintenance teams to ensure a better longevity of the equipment.
Step 6: Improving through feedback
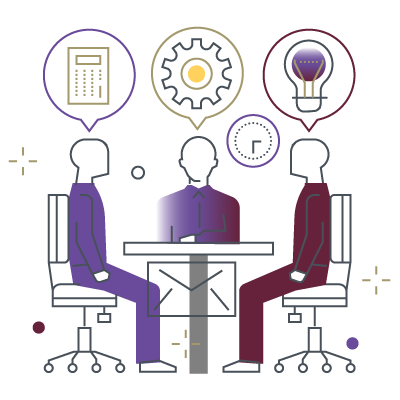
Some failures are easily detectable, others more complex to detect. Feedback is key in predictive maintenance as it continuously improves the model. As weeks and more exceptional outages progress, predictive modeling strengthens and detects increasingly complex failures. Several trades are mobilized to transform a failure incured into a detectable failure but the benefits are quickly visible.
To have data near its source, in a centralized and secure space, and avoid latency processing times, an Edge Computing solution is particularly suitable.
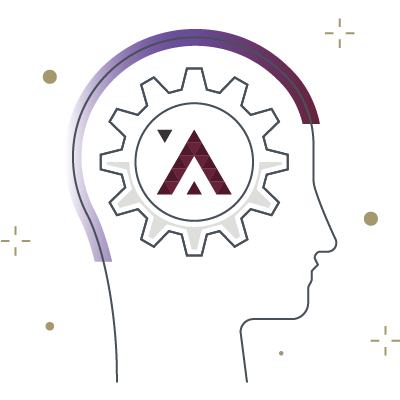
Feedback
It is necessary to be aware that the detection of failures from a modeling point of view is sometimes different from the point of view of the human. A maintenance technician can see “with the naked eye” that something is wrong, but it is difficult to transpose as it is in an algorithm.
We could easily imagine a camera and a detection algorithm. Environment and components often skew images (luminosity, pixel sensor size, etc.). Some failures will be detected in a similar way between what the human being does and the algorithm, others will not.
That’s why machine learning is a great support for predictive maintenance. These technologies will allow the system to learn to recognize new events and failures when they occur, in real time and continuously. There is a real complementarity to human analysis here.
Alexandre Langlais, CEO PERF’ACTOR
It offers many benefits and cases of use
Preventing malfunctions
By accurately and in real-time detecting anomalies and symptoms of equipment wear, predictive maintenance can anticipate failures. The company can then plan maintenance operations or repairs and thus maintain production without unexpected shutdowns. For example, infrared thermography (IR) solutions will detect and diagnose problems in mechanical equipment, electrical systems or boiler plumbing.
Reversing the logic of maintenance
Another advantage : agility. Indeed, predictive maintenance takes into account the context of the machine’s use and triggers maintenance actions if necessary. Thus, it preserves the maintenance of the facilities in operational condition. Predictive maintenance is said to « move from a logic of pushed flows to a logic of pull flow ». That is, it is the current state of the asset that triggers an intervention or not, not a predefined timetable. In practice, it is the role of the sensors to record usage data and transmit it to servers in order to analyze in real time the performance and the condition of the installations.
Anticipation and agility have the direct consequence of improving profitability and performance through cost and time savings, benefiting the company’s results :
- Reducing unplanned production shutdowns
- Decrease in spare parts inventories
- Lower repair and maintenance costs
- Improvement of the overall rate of return on equipment
- Increased product quality
SNCF has decided to deploy IoT sensors throughout its network. According to their CEO Guillaume Pépy, this predictive maintenance strategy will have saved SNCF nearly 537 millions euros between 2015 and 2020. These sensors also improve safety by preventing minor incidents from becoming serious.
It is important to note that most companies already have instrumentation that can be used for performance tracking and supplemented with inexpensive IoT sensors to meet predictive maintenance requirements. Another advantage is that predictive maintenance strengthens and enriches communication between technicians and analysts.
An improved quality of service
If we go on the side of suppliers of industrial facilities or maintenance services, this new technology can can improve customer satisfaction.. Because the supplier only intervenes when signals emitted by a machine reflect a likely failure in the short term. Let’s take the example of Schindler or ThyssenKrupp which decided to to set up sensors on their elevators.. Data that have been collected and analyzed about temperature allow them to « identify the maximum number of door openings before the near certainty of a failure » . Their customers are finally sure to have an elevator that works all the time.
The main tools for predictive maintenance are :
-
infrared thermal imaging
- vibration analysis to quickly identify causes of wear
- detection of ultrasounds that show cracks or bad welds
- liquid analysis to detect leaks and the presence of particles or to measure oil levels
- emission tests (e.g. waste gases) that reveal a lot about the state of the machines and highlight your environmentally friendly approach
Also, the installation of a digital twin allows you to enjoy all the advantages of predictive maintenance.
Aligning predictive maintenance with business strategy
Before deploying this technology, one must consider its relevance and potential gains because the initial investment is significant :
- diagnostic and surveillance equipment
- training of the factory staff
- data management
- possible hiring or training of analysts (data analyst, data scientist…)
Preventive maintenance will always be preferred in situations where there is a risk of human life.
Like any major change, the use of complex digital technologies requires a new mastery to acquire, the involvement and collaboration of the teams.
Predictive maintenance works very well if it is targeted. It is much more complex when the company wants to apply it on a large scale.
Companies often make the mistake of focusing on the result without thinking upstream about the strategy adapted to their dimension. Knowledge of business issues is also at the heart of a successful deployment of predictive maintenance.
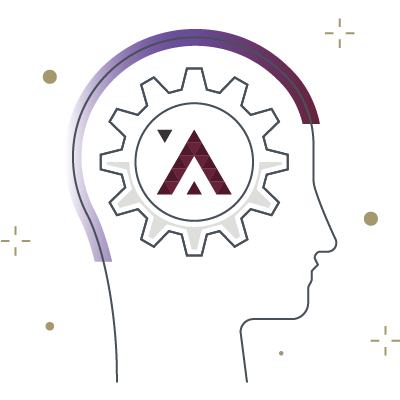
Feedback
To ensure the strategic alignment of your predictive approach, it is recommended to follow the following steps :
- Question the relevance of the project with respect to the company’s strategy and measure its long-term earnings and profitability.
- Do an audit of the production line, the different equipment, human resources and skills available and to be expected.
- Ensure end-to-end connectivity between the sensors and the back-end server: the reliability of this connection indicates the level of confidence to be given to the system’s predictions.
- Make sure the data collected is in line with the objectives you are looking for.
- Determine failure prediction models by combining data analysis, business expert opinion and experimentation with these models.
The most effective use of predictive maintenance depends on how you apply it to help you predict and avoid breakdowns, not just react to them. Also, it is interesting to use an evolutive solution that makes it possible to easily move to a higher dimension.
Alexandre Langlais, CEO PERF’ACTOR
Your industrial performance lever 4.0
In view of its many advantages (ROI, performance, agility…), this modern industrial maintenance technique has become established in factories.
Companies realized that through predictive maintenance, they could reduce outages, avoid unexpected events and downs and down the production line.
As a direct result, customer service is improved: predictive maintenance contributes to the delivery of products in accordance with the quality, prices and deadlines agreed.
In 2016, investments in predictive maintenance were already worth $9.1 billion globally (source : ABI Research). Also, according to McKinsey, predictive maintenance will save companies $630 billion by 2025.
In the age of Industry 4.0, predictive maintenance is one of the main levers of industrial performance for companies.
Ready to go for your predictive maintenance ?
✔ An expert of the industry of the future is listening to you for 45 minutes.✔ A 360-degree analysis to describe your level of maturity on 12 dimensions✔ Personalized advice to boost your competitiveness✔ Concrete cases adapted to your business✔ A visual and synthetic synthesis in your email inbox
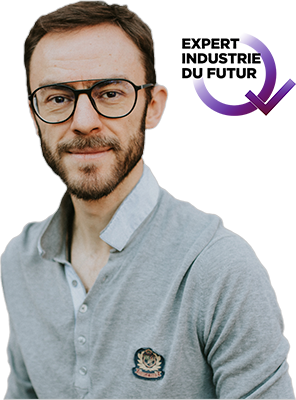
Alexandre Langlais, CEO PERF’ACTOR